Using a blockchain structure, community members can vote on what data should be included in the meta-analysis for any given topic.
ARUP Healthcare Advisory Services team members Ryan Nelson, PharmD, medical director of Precision Medicine, and Erik Forsman, senior data consultant, have built the basis for an open access database that could change the way researchers perform meta-analyses. In the case of pharmacogenetics, it will accelerate physicians’ access to up-to date information in this emerging and rapidly changing discipline so that they can make better-informed clinical decisions about which drugs (e.g., chemotherapy agents, antidepressants, etc.) are most likely to be effective for their patients based on their genetic makeup.
Question (Q): Tell us about why you started this project.
Ryan Nelson (RN): As clinicians, we can’t do our jobs without researching and validating our hypotheses. Sometimes, though, published studies contain insufficient sample sizes to adequately answer the question being asked. To increase sample sizes and measure the broader effect of a specific medical question, researchers compile the results from a group of individual studies that are asking the same question using similar methodology. Then they meta-analyze the conglomerate results. That’s where the problem occurs. These meta-analyses are usually done by small author groups every three to four years, and they all say they’re using the same guidelines to determine whether a study should be included in the meta-analysis. But what we’ve found when we look at individual meta-analyses on the same topic is that they don’t agree precisely on which studies should be included, which leads to scientific discord rather than consensus.
Q: How do you fix that problem?
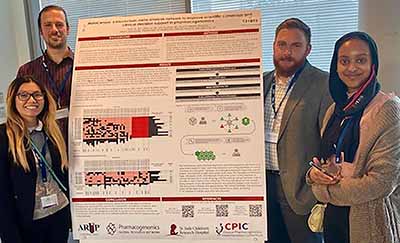
Erik Forsman (EF): To start, we need to expand the network of researchers who develop the protocol that determines which papers should be included in a meta-analysis. By expanding the network of researchers contributing to the same meta-analysis, we reduce the risk of misrepresenting scientific consensus on the research question. We then need to expand access to the results of the meta-analysis. We have chosen to decentralize the data and make them accessible to everyone. From a data analytics perspective, the data are currently centrally located within each study. You can also look at these data as siloed because you aren’t always going to have access to every available study. When researchers are able to access the data from the studies on a more regular basis and interact with a community of like-minded peers, then a consensus can be reached more quickly and accurately.
RN: We’re starting with pharmacogenetic research, and we’ve chosen two separate gene-drug pairs related to preventing toxicity in chemotherapy. One has a guideline from the Clinical Pharmacogenetics Implementation Consortium (CPIC), DPYD genotype-guided fluoropyrimidine dosing, and one is without a CPIC guideline—UGT1A1 genotype-guided irinotecan dosing. And what we’ve done is taken all the individual studies from the different published meta-analyses for these two gene-drug pairs and abstracted the data from them. Now we’re taking the data from these analyses and putting them into a network structure. We chose to do it this way so that we can compare levels of evidence and see if there’s a reason why one gene-drug pair has a CPIC guideline, but not the other, and support the development of guidelines along the way.
Q: What does this structure look like?
RN: We thought it might be interesting to use a fragmented approach that allows the data to be stored and owned by the public at large through a blockchain network.
EF: Blockchain is a data structure of distributed databases shared among a network of independent parties. The beauty of blockchain is that the data are owned by everyone instead of one person, so everyone will always have access to the same data at the same time. Even if they interact with the data, it’s always going to be the same interaction at the same time, so no one person is able to make changes without consensus voting by the network of voting community members. And every change that IS made is logged, so as new research comes out, it’s easy to see where the data changed and an updated consensus was reached.
Q: Will people mine the data, like with cryptocurrency?
EF: No, this is nothing like cryptocurrency. We’re simply using the same technology because it offers a self-maintainable system with a complete history of every transaction, or in this case, every vote.
RN: We’re creating a community, probably several in the long run, of researchers with aligned clinical foci who can help create protocols to definitively determine which studies to include in a meta-analysis for a specific topic. They can then vote on whether the evidence from the meta-analysis supports clinical actions based on the data. Then we can make that information digestible for physicians to help them determine which type of testing they should be ordering based on the research that’s been done.
Q: How are you gathering this community?
RN: The pharmacogenomics community is a rather tightly connected community. Every couple of years a conference is held that’s focused on pharmacogenomics. The CPIC and Pharmacogenomics Global Research Network (PGRN) conference was held earlier this month. We presented a poster on this project there and received a lot of positive feedback and interest in determining how to engage with it. What we now need is to recruit beyond our pharmacogenomics community; we need medical oncologists unfamiliar with CPIC and PGRN to also review and vote on these data. We need biostatisticians who hold no particular interest in any one topic to review the data based on sound study design. And we need members from leading institutions who develop clinical guidelines on related topics to contribute their feedback and vote as well. We intend to leverage our network of colleagues to grow the project.
Q: So, what’s next?
EF: Testing, testing, and more testing. We’ve got the network’s skeleton built out, but we really need to test it out to make sure that everything is working the way we want it to on the back end. Then we can begin to work on how the community will interact with the data through a website or application.
RN: We also need to recruit the founding members of the network. These individuals will refine the meta-analysis protocol, the consensus threshold for study inclusion, and the framework for methods to leverage the data beyond providing a robust, up-to-date meta-analysis. From there, we hope to gain support from related communities that have already abstracted data for meta-analyses that the community could review for inclusion. We don’t want to reinvent the wheel here; we just want to improve its traction and reduce its rolling resistance. There is a strong community of clinicians and health scientists who have advanced and will continue to advance the field of pharmacogenomics. We simply want to provide a tool to accelerate and unify our efforts.
Those interested in learning more about this project or participating in this community can visit aruplab.com/consensus to apply to become voting members and review the poster presentation given at CPIC.
Jennifer Sanda, jennifer.sanda@aruplab.com